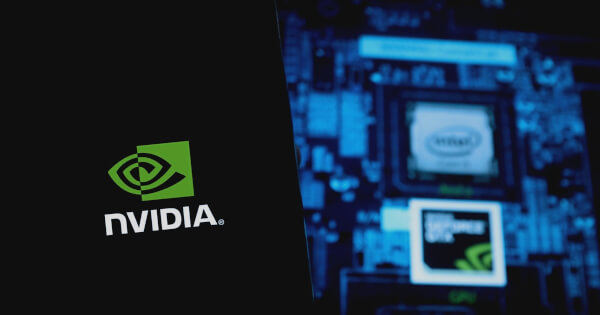
Lawrence Jengar
Nov 22, 2024 16:35
AI technology, Eye-AD, analyzes retinal scans to detect early signs of Alzheimer’s, providing a non-invasive and rapid screening method, according to NVIDIA’s study.
In a groundbreaking development, artificial intelligence (AI) is being leveraged to detect early signs of Alzheimer’s disease through retinal scans. The study, reported by NVIDIA, introduces a deep learning framework named Eye-AD, which analyzes high-resolution retinal images to identify subtle changes in the vascular layers of the eye that are often linked to dementia. This innovative approach offers a rapid, non-invasive screening method that could significantly enhance early detection and treatment of cognitive decline.
The Importance of Early Detection
Alzheimer’s Disease (AD) currently affects over 50 million people worldwide, and the number is expected to rise as the global population ages. Early detection is crucial for improving patient outcomes and quality of life, enabling timely clinical interventions to slow disease progression and allowing families to plan for long-term care and support.
Retina: The Window to the Brain
The retina, often referred to as a ‘window to the brain,’ shares embryonic origins with the brain. Research indicates that changes in the retina’s microvasculature—tiny blood vessels—are often associated with cognitive decline. Traditional detection methods such as MRI and spinal fluid analysis are costly and invasive, making Eye-AD’s non-invasive approach particularly promising.
Technical Advancements of Eye-AD
Developed by researchers, Eye-AD combines a convolutional neural network (CNN) to extract features from retinal images with a graph neural network (GNN) to analyze relationships within and between retinal layers. It uses Optical Coherence Tomography Angiography (OCTA) images to visualize blood flow and vascular details, identifying clinical biomarkers to predict early-onset Alzheimer’s Disease (EOAD) and mild cognitive impairment (MCI).
The model was trained on 5,751 OCTA images from 1,671 patients using PyTorch on a workstation with four NVIDIA GeForce RTX 3090 GPUs, which significantly accelerated the training time and processing efficiency of the high-resolution images.
Performance and Future Prospects
Eye-AD demonstrated remarkable accuracy, outperforming other models in detecting EOAD with an AUC (Area Under the Curve) of 0.9355 on internal datasets and 0.9007 on external datasets. While its performance for MCI detection was slightly lower, it still achieved an AUC of 0.8630 internally and 0.8037 externally. The study highlighted the deep vascular complex in the retina as a key biomarker for predicting early disease.
The researchers emphasize that Eye-AD represents a substantial advancement in dementia detection, with potential for widespread use in cognitive health assessments. Future efforts will focus on validating the model across diverse populations and integrating it with other diagnostic tools to assist doctors in clinical practice.
The Eye-AD source code is accessible on GitHub, providing an opportunity for further development and research in this promising field.
For additional insights, read the full study on Nature.
Image source: Shutterstock
Be the first to comment